Mohammed El-Kebir Hunts for Cancer’s Evolutionary Trees
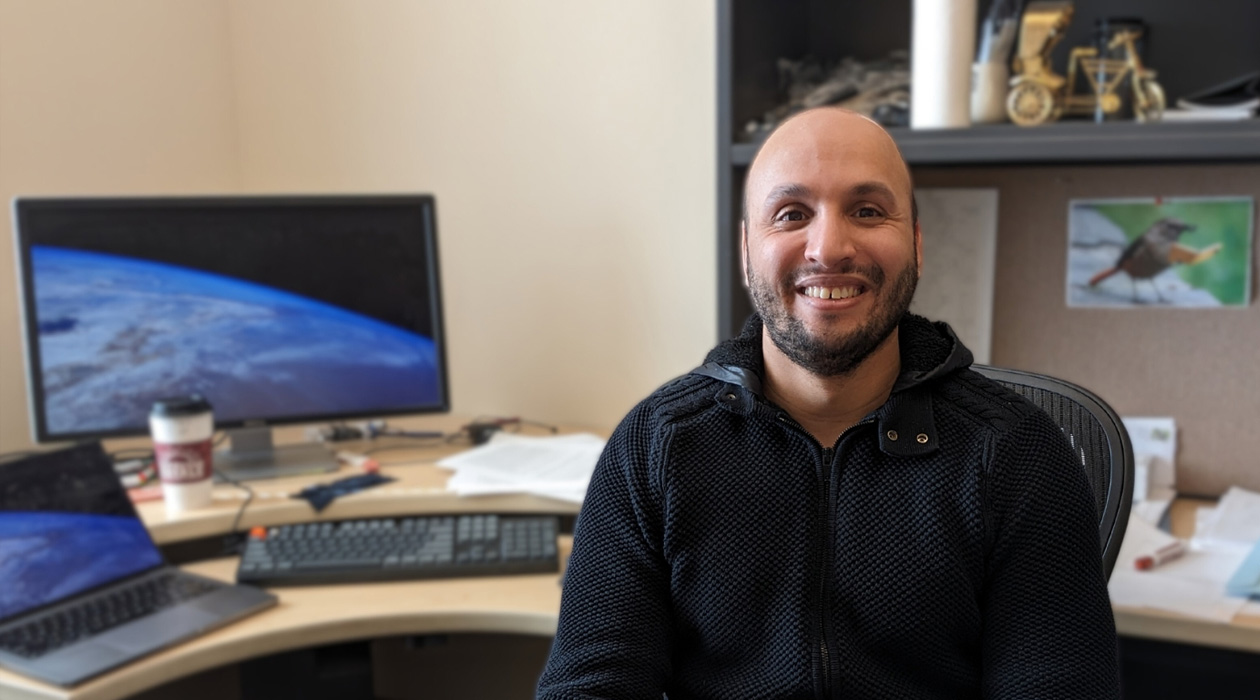
Mohammed El-Kebir is a professor of computer science. / Jonathan King
Mohammed El-Kebir (CAIM/IGOH) is an assistant professor of computer science researching cancer genomics, who investigates combinatorial optimization algorithms for problems in computational biology, and his lab has made major advances in the theoretical foundations of cancer phylogenetics and methods for the estimation of cancer phylogenies from sequencing data of tumors.
In other words, El-Kebir’s lab develops mathematical models to understand how cancer evolves.
What might initially sound like a fantastic scenario for artificial intelligence (AI) to the untrained eye, El-Kebir’s expertise as a computational biologist holds a different vantage point, where he analyzes data in novel ways to discover new biology.
“It’s important to understand that AI will not be the solution for every problem and every question we have in research,” says El-Kebir. “AI works best when we have ground truth, labeled data. It works when it’s a classification problem—is it healthy or diseased tissue? Did the tumor respond to the treatment or not? Those are labeled outcomes. That is where AI works well,” says El-Kebir.
For example, in a situation such as cancer imaging where there are clear ones and zeroes, researchers have ground truth data in which they can apply their AI tools. Not so with the problems that El-Kebir’s computational biology lab faces. Computational biologists are exploring the frontier of biology, foraging among the wild edges of data.
Therefore, El-Kebir’s team must design their own tools—customized algorithms—rather than rely on general purpose machine learning models.
Like sitting down to boxes of mixed-up puzzles without any photo evidence to guide them, El-Kebir’s team seeks to reconstruct the past to understand how cancer evolved. They hunt for the evolutionary tree, looking for the roots and branches where cancer mutations grow.
“Imagine this mixture as a kind of puzzle,” says El-Kebir. “It’s not just one puzzle though. In this abundance of clones, each clone corresponds to a different puzzle. Imagine ten of these puzzles, and for each puzzle you have maybe five to twenty boxes of pieces, but the number varies for each box and you have to take all those pieces and mix them together and then you’re told: ‘Go make all those puzzles! But there’s a catch. You don’t have an outline or an image to match.’ That’s the game we are playing with bulk sequencing data as we try and reconstruct cancer evolution.”
To reconstruct this evolutionary tree model of cancer, El-Kebir’s lab takes genomic sequencing data from a tumor that they have obtained through clinical collaborations. They study two types of data. First, bulk DNA sequencing (involving one or more biopsies from a single tumor), which sequences all cells in the biopsy simultaneously. From this, they obtain short DNA fragments of length about 100 nucleotides, originating from different cells. These different cells correspond to different clones, with each clone providing a unique set of mutations.
The second type of data El-Kebir uses is single-cell DNA sequencing. With this data they are looking at single cells and profiling DNA of an individual cell. “It’s simpler—a single puzzle,” says El-Kebir. “But it’s sparse, so there are lot of missing puzzle pieces. And some puzzle pieces have an error, so there could be mutations in that box of pieces that shouldn’t be there. So, you must deal with the limitations of the data before you can get the evolutionary tree you care about.”
If this sounds like a herculean feat, it is indeed a challenge. But it’s a challenge that motivates El-Kebir and his computer science team who thrive when faced with difficult, novel computational problems such as the puzzles presented in the study of cancer’ evolutionary history and mutations.
“One of the reasons cancer is so hard to treat is because of intratumor heterogeneity,” says El-Kebir. a tumor, the cells are not homogenous. When you apply a treatment to the tumor, maybe only a subset of the clones responds, and those clones that don’t respond could then take over the population. That’s why you get treatment resistance. The tumor itself is a result of cancer evolution.”
This “tree” is the puzzle that El-Kebir’s lab seeks to reconstruct, including the order in which the mutations were introduced. What happens then once the puzzle pieces are put together?
“That’s where AI comes in,” says El-Kebir. “With this labeled data in hand, we can partner it with a drug response. This tumor responded to this drug, and this tumor did not. This is what happened in the ‘tree.’ This helps us predict or learn what will happen in response to different types of therapy.”
This information also helps predict future tumor evolution, which has important implications for personalized medicine, or patient-specific therapies.
“The reason therapies fail is because you get resistance,” says El-Kebir. “And you get resistance because of all this heterogeneity in the tumor cells. There are all these different clonal populations. If you know the populations present, it will help you think more carefully how to treat them or contain them because complete eradication may be counterproductive. Just like with antibiotics, you don’t want to use up all the drug options immediately. There’s the first line and then the second line defense. You want a backup plan if the first line fails. If you try to aggressively eradicate the tumor you may end up with a small population that didn’t respond, because they were not as fit as other clones in the tumor. But if they are given the opportunity to take over, they may go rogue. One strategy might include looking at the cells that do respond to therapy and partitioning them into two different groups with alternating drugs to maintain an equilibrium. That’s the game you must play.”
One might wonder how a computer scientist at a basic science cancer center comes to these conclusions. El-Kebir isn’t surprised by that curiosity. In fact, he has devoted himself to studying the data and he’s learned to see the patterns. “I have these perspectives because I have been looking at data for a long time—data before and after treatment. I see what happens. I see the resistance. Patterns emerge. I’ve seen the nondominant clone become dominant. The data don’t lie,” says El-Kebir.
El-Kebir notes the unique position for CCIL researchers to address cancer problems with the help of AI. “We are at the forefront of fundamental science of AI on our campus. There’s a great opportunity to connect with those on the application side, as we develop the science,” says El-Kebir, who observes close-up the rapid growth of our understanding of AI. “I see the implications for basic cancer science that can be translated to the clinic. Cancer immunotherapy—personalized medicine—is one of the prime areas where AI will help us leverage individual patient’s immune systems to clean up cancer.”
And El-Kebir is hopeful about this future as his lab devotes themselves to their work among the edges of fundamental science that will yield fruit for tomorrow’s cancer patients in the clinic.
Mohammed El-Kebir is an Assistant Professor in the Department of Computer Science and affiliate of the National Center of Supercomputing Applications at Illinois.